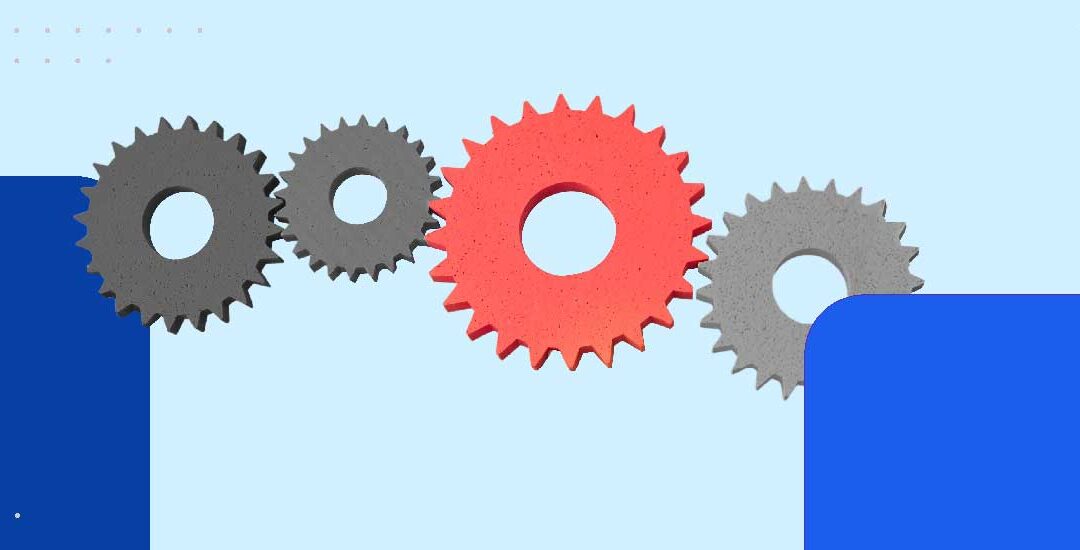
Applications for Machine Learning in Different Sectors
Machine learning can streamline processes and provide data-driven insights.
Machine learning (ML) uses algorithms to imitate the way humans process information. Namely, they do this by gradually improving their accuracy by processing more and more data and assessing the outcomes of their analyses.
Machine learning software can do this process and analyze large amounts of data quickly. It can find trends, patterns and insights that humans might miss or could find only after a lengthy research process.
Accurate, data-driven insights from a large amount of data points can play a significant role in decision-making within a company. With consistent and accurate insights, companies can make more informed decisions and also get analysis of the results of their choices in near real-time. This information can help firms gain an edge over their competitors.
Companies that do not utilize this technology will be in the minority. According to a 2021 McKinsey report, nearly 56 percent of surveyed companies reported using artificial intelligence tools like ML for at least one function. Those that don’t follow along will risk falling behind without the analysis necessary to make informed decisions in competitive marketplaces.
This competition is also all-encompassing. A variety of industries have been and continue to be affected by machine learning, so let’s take a look at some examples.
Machine learning in finance and accounting
Machine learning has multiple uses in the finance industry. In 2022, chipmaker Nvidia claimed 75 percent of companies used at least one high-performance computing (HPC) tool, including machine learning and deep learning software, in their operations.
The finance industry relies heavily on analysis for decision-making, so machine learning can play a sizable role in everyday operations.
Before ML and algorithm-powered analysis came along, banks, lenders, investment firms and financial service providers arrived at insights manually. This labor-intensive process took time and created work stress for employees. Human error could complicate these analyses and lead to potential problems. Such mistakes are also an important factor in auditing and assurance work. Automated software can speed up the auditing process and ensure accuracy.
Here are four examples of how machine learning can streamline financial industry operations:
- Fraud detection software can find patterns common in financial fraud and immediately flag activity for further review. These warnings can be invaluable for lenders, payment processors and other financial services companies.
- Risk management software allows companies to find and define risks, constantly update their risk profile based on new data and adjust to account for evolving financial markets, regulatory changes and trends.
- Algorithmic trading involves using machine learning to find trends in financial markets, create forecasts about future price movements and purchase or sell securities or other assets based on ML analysis.
- Financial advisory services can use machine learning tools to weigh the financial needs of clients and make suggestions or offer investment products based on automatic ML analysis. These services are less costly than working with a human advisor.
In all these examples, ML frees employees to work on higher-level tasks.
Machine learning in manufacturing, transportation and logistics
Comparisons with other industries outside accounting and finance reveal meaningful learnings. For example, machine learning can optimize the manufacturing process. ML software can offer a holistic view of the entire manufacturing process — from the supply chain to conditions in production facilities to materials and inventory. Machine learning tools can analyze data from all these sources and find ways to optimize the entire process and increase cost savings.
Here are three specific areas where machine learning is especially useful in manufacturing:
- Predictive maintenance of machinery can reduce downtime and overall repair costs. ML software can use data and information from sensors or embedded systems to predict repair needs and create an optimal schedule for preventative maintenance.
- Quality control is essential to manufacturing. ML software can monitor quality beyond the finished product. It can analyze materials, shipping and storage methods and production techniques to find the source of quality lapses and steps toward quality improvements.
- Supply chain optimization is challenging for manufacturers, especially those relying on many different materials. Machine learning can forecast materials needs, predict potential supply chain disruptions and help optimize inventory to avoid over or understocking certain materials.
In all these examples, ML helps enhance overall operational efficiency, limit production delays and maximize productivity.
Manufacturers rely heavily on the logistics and transportation industries for raw materials and shipping finished products. Machine learning also impacts these sectors.
- Demand prediction tools allow logistics planners and fleet managers to prepare for upcoming surges in shipping orders. They can have the vehicles, drivers and warehouse staff on hand to deal with the increased demand.
- Route optimization software uses machine learning algorithms to map the most expedient routes for vehicles or goods. These planning tools can limit fuel use and driving time to help transportation companies save on operating costs and ensure clients get their goods as quickly as possible.
- Fleet management systems use ML to predict maintenance for vehicles, schedule drivers according to demand and safety and rest regulations, and re-route vehicles experiencing delays.
In addition to cost savings and increased efficiency, ML can help streamline logistics operations. This dynamic facilitates organization and aids clear communication with employees and clients.
Integrating machine learning in business
Machine learning can aid decision-making, reduce workload, limit manual tasks that cause burnout and eliminate instances of human error.
Machine learning tools can be an investment — both in terms of cost and the time it takes to make operational changes. Implementation is a step-by-step process and careful planning can ease the process of integrating machine learning into everyday business operations.
Here are four considerations for companies integrating machine learning:
Assessing business needs and goals
Companies need to apply machine learning to meet their specific needs. The first step in integrating these tools into operations is to analyze current processes.
Those in charge of integration can find areas where employees are spending a lot of time on basic tasks, where errors are common or where decision-making is uncertain. Machine learning can assist with these tasks, cutting work hours and costs and increasing accuracy and efficiency.
Machine learning integrations should also support objectives and current business goals. These tools can enhance operations, but companies should not alter their plans to accommodate machine learning tools.
Collecting and preparing data
The quality of data can limit the benefits of machine learning. The algorithms used in machine learning systems require good training data. Without it, they are unable to make accurate predictions. Data analytics tools need information that addresses the specific problem they are trying to solve or the processes they are attempting to streamline.
Machine learning software is more accurate with larger datasets. It can adjust to outliers and subtle trends with enough information to input. ML programmers can look for incorrect data, duplicate examples or mislabeled information, which will confuse the software as it tries to improve accuracy.
Selecting the best machine learning tools
Machine learning provides insights or automates tasks. Ultimately, its purpose is to help businesses solve specific problems. No matter your industry, you should research a variety of tools and pick one that will provide answers for your company’s specific needs.
If a company works with third-party providers offering machine learning tools on cloud-based platforms, it needs to consider risk management. Software-as-a-service (SaaS) providers should be reputable and have quality security in addition to offering the functionality the company needs.
Implementing and scaling machine learning solutions
The first step in implementation is to test the machine-learning solution to see if it provides the desired results. Once this testing phase is over, the company can scale up the use of the software.
Cloud-based solutions are often the ideal choice because they are scalable. With physical on-site servers, companies need to add hardware when they want to increase reliance on specific software. Cloud-based software does not require this physical expansion. Companies can simply upgrade their service to meet the increased memory and speed needs.
Businesses do not need to build machine-learning solutions from the ground up. Reputable SaaS and platform-as-a-service (PaaS) vendors can provide customizable ML solutions that companies can set up and scale to fit their needs. These partners can simplify the planning and implementation process and allow the company to add or expand ML tools as they grow.